Unique Registration Number:334
Innovator Name:
Shri Sourabh Naik Khandolkar.
Shri Swapnil Namdeo Gawade.
Shri Amey Gaonkar
Smt Pradnya Mhalseka
Contact No: +919657886339
Contact Email: sourabhnk61@gmail.com
Project Objective:
To detect and classify different stages of Diabetic Retinopathy using screened retinal fundus images of the diabetic patients with the help of Machine Learning
Abstract:
Diabetic retinopathy is an eye complication that occurs only among diabetic patients and causes partial or complete permanent damage to their vision. Which is why timely detection and treatment being necessary to prevent further complication. Most of the time the time window for treatment is missed as the manual screening and detection takes around 3 weeks, also the results of manual diagnosis of DR aren’t always accurate. Hence there is a serious need to reduce this 3 weeks’ duration so that the patient and the doctor can start the treatment rapidly and scout for a more accurate result. We plan to accomplish this with the help of machine learning wherein the screened retinal fundus images would be fed to our machine learning model and it will classify if the particular eye has DR or not. If yes, then the level of DR would also be classified weather it is mild or moderate or severe based on the characteristics from the images. Hence this process will reduce the duration to few hours instead of few weeks which the manual process requires. Our process also increases the efficiency as the manual method might have some irregularities as they are physically scanned by ophthalmologist. This way the patient as well as the eye specialist can start timely treatment as the time window for the treatment gets increased. This will also reduce the cost for the patient and save the time of the doctor.
Project Outcome/result/findings:
The dataset of the screened retinal fundus images consisting of 35000 images has been fetched and preprocessing using different image preprocessing techniques like Gaussian blur and auto cropping is been accomplished. The user interface and web app is ready and the training of the machine learning model(CNN) is underway. The preprocessing techniques give successful results
Innovative Approach:
We train a machine learning model (ResNet50) with the help of 35000 sorted retinal fundus images and later test it with another set of mixed fundus images to classify the Diabetic Retinopathy disease and its severity levels. We then fine tune the final layers ML model to achieve optimal accuracy in classification of the disease and its severity levels.
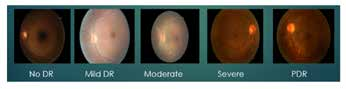